www.magazine-industry-usa.com
09
'20
Written on Modified on
Maturity self-assessment for Industry 4.0
One of the biggest questions posed by manufacturers looking to transform their operations with artificial intelligence is: How do we know if we've got the data for this? It is a really good question. Machine learning is largely dependent on the ingestion of data - and not just any data. This article looks at the complexity that surrounds large industrial operations and the type of data manufacturing plants are probably already collecting. We also take a look at supporting the systems and infrastructure required.
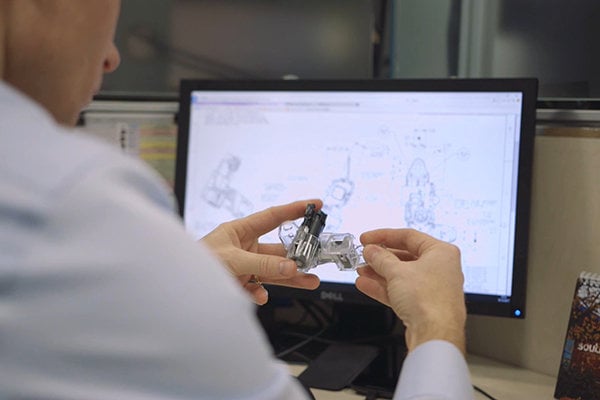
It starts with quality
In modern production environments, there are many places to measure the pulse of your operation: from takt time on your production line, to the size of your goods-receiving warehouse, and then to scrap and rework rates, and even the cost of non-quality. All of these have a direct impact on your business's efficiency, and it is important to understand the dynamic interplay between these variables.
At some point in your production process, a quality-deviation occurs and this quality event is a function of all the processes upstream of the event. This has an impact on all the processes downstream of the event. If uncaught, this quality deviation propagates all the way to your customer and that becomes an even more difficult conversation to have. So the most critical data, and minimum requirement, is to have some measurement of quality and where in your process the quality measurement is made.
Some organisations use the simple differentiator of "internal quality" (where the quality inspection team has identified a defect and quarantined it) and "external quality" (where the incoming inspection at the customer has identified the defect). In processes where there are different defect types, it is also very valuable to have the defect type, but this is not a minimum requirement.
Following the process: identification and traceability
To gain useful insights into your entire process the quality result needs to be connected to the conditions that either enabled a good quality result or led to a poor quality result. To achieve this process mapping, there are two key requirements:
-An identifier (usually a part ID or batch number); and
-A record of where in the process the component is.
The International Organization for Standardization (ISO) maintains a suite of standards related to quality management, known as the ISO 9000 family. ISO-9001 details the requirements for quality management systems (QMS) and this standard has several minimum requirements for the identification and traceability of business. These requirements are far in excess of what is required to track the quality result through a process, but are a great yardstick to guide future quality system implementations. However you achieve it, the next minimum requirement is the traceability of an article or batch through your process.
Process control: set-points and targets
Controlling your manufacturing process is undoubtedly happening now because your process isn't just running on random. It is highly likely that if the parameter has an impact on the quality result, it is already measured and controlled. There are many parameter control paradigms: from simple closed loop PID control, through to more advanced black-box Model Predictive Control (MPC). All of these compensatory control regimes have an impact on the shape of distributions of the control variable - as do the parameters of the transfer function describing the underlying process.
The difficult part of this problem is the determination of the complex interplay between parameters: the extent to which a small change in one impacts adjacent parameters and how the change cascades down through subsequent processes. The complex question is: how is the control target set and what method (if any) is being used to compensate for the inherent variation in all the other process variables?
A holistic representation: combining quality, traceability and control
This is the hardest part of realising value from Industry 4.0 installations. Combining these three different data sources is crucial to creating a representation upon which an AI system can learn how process parameters influence quality and process yield. This is largely based on mapping out the process but is also heavily dependent upon capturing a description of the variability and randomness of each variable. It is unlikely that manufacturers have a view of their data that meets these requirements so this is one of the key pieces of work that we do at DataProphet.
Running with AI
DataProphet uses machine learning to understand how a plants process variables interact and combine. Machine learning is amazingly well suited to this problem and the output is a control plan that can enable production teams to fine tune processes to reduce defects and eliminate scrap.
Dr Michael Grant, CTO, DataProphet