www.magazine-industry-usa.com
08
'24
Written on Modified on
MEDIAWORLD
GENERATIVE AI: TOOLS, MODELS, & APPLICATIONS
K.A. Gerardino explores Generative AI, where precise engineering meets limitless creativity to reshape intelligent systems.
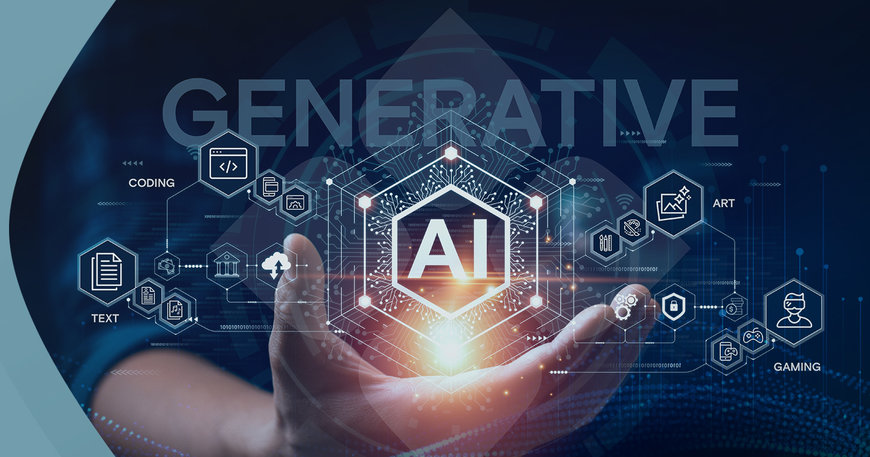
Generative AI is an interesting area of study that involves the creation of machines capable of producing content, such as images, text, or music, that is virtually indistinguishable from content created by humans. This technology relies on sophisticated algorithms and models to generate new, realistic data based on patterns learned from existing data.
In this comprehensive exploration, we discover the advanced tools, intricate models, and real-world applications propelling the evolution of Generative AI. Ready yourself for an in-depth analysis of algorithms and frameworks, uncovering the inner workings that position artificial intelligence at the forefront of innovation. Join us as we navigate the complexities of this transformative field, where precision engineering converges with boundless creativity to redefine the very fabric of intelligent systems.
The global generative AI market size reached US$12.3 billion in 2023 and is expected to hit US$58 billion by 2032, demonstrating a growth rate (CAGR) of 18.81% during the period of 2024-2032, according to IMARC Group. The increasing volume of generated data, the advancement of cutting-edge machine learning techniques, the expansion of the information technology (IT) sector, and the growing adoption of products across various industries are among the key factors driving the market forward.
Embarking on a comprehensive exploration of Generative AI reveals a dynamic ecosystem teeming with innovation, where tools, models, and applications converge to redefine the very fabric of artificial intelligence. Let us delve into the intricate layers of each key aspect, unravelling the nuances that collectively contribute to the transformative nature of this growing field.
Here are some key aspects of generative AI, including tools, models, and applications:
Generative AI Tools
- OpenAI's GPT (Generative Pre-trained Transformer): GPT-3, the latest version, is a powerful language model that has been widely used for various natural language processing tasks. It can generate coherent and contextually relevant text based on prompts provided to it.
- DeepDream: Developed by Google, DeepDream is an image generation tool that utilizes neural networks to enhance and modify images. It highlights and exaggerates patterns and features in images to create visually stunning and sometimes surreal results.
- StyleGAN (Generative Adversarial Network): StyleGAN is a popular model for generating realistic images. It excels in creating high-quality, diverse images with customizable styles. StyleGAN2, an improved version, has been widely adopted in the creation of deepfake images.
- GANs (Generative Adversarial Networks): GANs are a class of algorithms where two neural networks, a generator, and a discriminator, are trained simultaneously through adversarial training. GANs are used for various generative tasks, such as image and video synthesis, as well as style transfer.
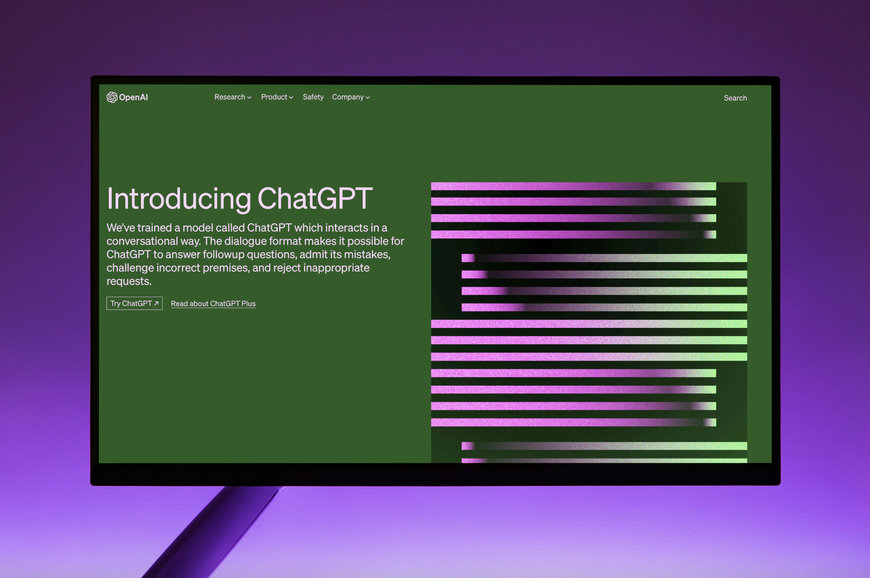
Photo: Andrew Neel on Pexels
Generative AI Models
- BERT (Bidirectional Encoder Representations from Transformers): Although initially designed for natural language understanding, BERT has been used for generative tasks as well. It understands the context of words in a sentence, making it suitable for generating coherent and contextually relevant text. Although initially designed for natural language understanding, BERT has been used for generative tasks as well. It understands the context of words in a sentence, making it suitable for generating coherent and contextually relevant text.
- VQ-VAE-2 (Vector Quantized Variational Autoencoder 2): VQ-VAE-2 is an advanced model for image generation. It uses vector quantization and variational autoencoder techniques to produce high-quality and diverse images.
Generative AI Applications
- Content Creation: Generative AI is used in the creation of digital content, including images, text, and music. This can be applied in various industries, from graphic design to entertainment.
- Deepfake Technology: GANs have been employed in the development of deepfake technology, enabling the creation of realistic-looking videos and images with manipulated content.
- Artificial Creativity: Generative AI is being explored as a tool for enhancing human creativity. Artists and designers use these models to inspire and assist in the creative process.
- Data Augmentation: In machine learning, generative models are utilized for data augmentation, creating additional training examples to improve the robustness and generalization of models.
- Drug Discovery and Molecular Design: Generative models are applied in pharmaceutical research to generate novel molecular structures for drug discovery.
While generative AI holds tremendous potential, it also raises ethical concerns, particularly in the context of deepfake technology and the potential misuse of generated content. Striking a balance between innovation and responsible use is crucial as this field continues to evolve.
Generative AI market growth factors
The generative AI market has witnessed significant growth in recent years, driven by several factors that contribute to its adoption across various industries. Here are some key growth factors for the generative AI market:
The generative AI market has witnessed significant growth in recent years, driven by several factors that contribute to its adoption across various industries. Here are some key growth factors for the generative AI market:
- Advancements in Deep Learning: Breakthroughs in deep learning architectures, such as Generative Adversarial Networks (GANs) and Transformers, have significantly improved the capabilities of generative AI models. These advancements have led to the creation of more realistic and sophisticated content, driving demand in the market.
- Increased Computing Power: The availability of powerful GPUs (Graphics Processing Units) and TPUs (Tensor Processing Units) has enabled the training of larger and more complex generative models. This increased computing power has played a crucial role in the development of state-of-the-art generative AI applications.
- Open-Source Frameworks and Tools: The availability of open-source frameworks and tools, such as TensorFlow and PyTorch, has democratized access to generative AI technology. This has lowered the entry barriers for developers and businesses, fostering innovation and expanding the overall market.
- Rise of Pre-trained Models: Pre-trained models, like OpenAI's GPT and various versions of StyleGAN, have gained popularity. These models, trained on massive datasets, can be fine-tuned for specific applications, accelerating the development process, and making generative AI more accessible.
- Diverse Applications Across Industries: Generative AI finds applications in a wide range of industries, including entertainment, healthcare, finance, and manufacturing. This versatility has contributed to its market growth as businesses explore innovative ways to leverage generative AI for various use cases.
- Increased Data Availability: The abundance of digital data available for training generative models has been a crucial factor in their success. Large datasets allow models to capture diverse patterns, resulting in more accurate and realistic outputs.
- Creative Content Generation: The demand for creative content, including images, videos, and text, in industries such as marketing, design, and entertainment has fueled the growth of generative AI. Businesses are leveraging these technologies to streamline content creation processes and deliver engaging material.
- Research and Development Investments: Increased investments in research and development by both private companies and public institutions have driven innovation in generative AI. This ongoing research contributes to the development of more advanced models and applications.
- Growing Awareness and Adoption: As awareness of generative AI capabilities spreads, more businesses are recognizing the potential benefits of incorporating these technologies into their operations. The growing adoption across sectors further fuels market growth.
- Regulatory Support and Ethical Considerations: Clear regulatory frameworks and ethical guidelines around the use of AI technologies, including generative AI, provide a supportive environment for businesses to invest in and deploy these solutions. Addressing ethical concerns ensures responsible development and use of generative AI.
The convergence of these factors is driving the generative AI market, and its continued evolution is expected to bring about further innovations and applications across diverse industries in the coming years.
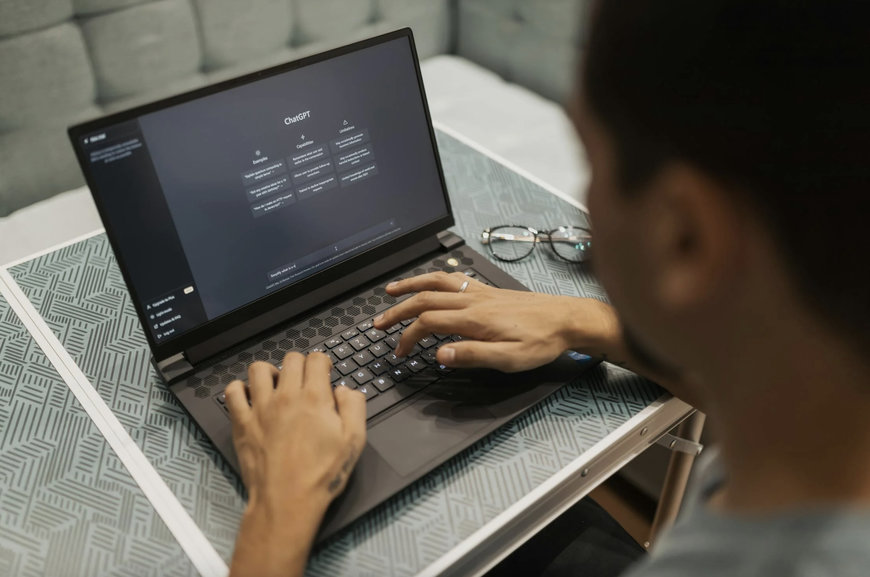
Photo: Matheus Bertellii on Pexels
Restraining factors in Generative AI
While generative AI has experienced substantial growth and advancement, there are also several restraining factors and challenges that impact its widespread adoption.
Generative AI models often require large amounts of data for training, which raises concerns about data privacy and security. The use of personal or sensitive data in training sets may lead to privacy breaches and ethical issues. The potential for misuse of generative AI, especially in the creation of deepfakes or other malicious content, raises ethical concerns. Addressing the responsible and ethical use of these technologies is crucial to mitigate negative consequences.
Generative AI models can inadvertently learn and perpetuate biases present in the training data. This can lead to biased outputs, reinforcing existing societal prejudices and discrimination. Ensuring fairness and addressing bias in generative models is an ongoing challenge.
Many generative AI models, especially complex neural networks, lack interpretability. Understanding how these models generate specific outputs can be challenging, limiting their trustworthiness in critical applications where interpretability is essential.
Training and running sophisticated generative AI models often require significant computational resources, including powerful GPUs and TPUs. This resource intensiveness can be a barrier for smaller organizations or those with limited computing infrastructure.
The legal landscape surrounding generative AI, particularly in the context of intellectual property rights and ownership of generated content, is still evolving. Clear regulatory frameworks are needed to address legal challenges associated with generative AI.
Generative AI models may face challenges in generalizing well to diverse and unseen data. Overfitting to specific patterns in the training data may result in poor performance on new and varied inputs.
Understanding and predicting the behaviour of generative AI models can be difficult. This lack of understanding may hinder the adoption of these models in critical applications where predictability is essential.
Building and maintaining state-of-the-art generative AI models can be expensive. This cost factor may limit the accessibility of generative AI technologies for smaller businesses and organizations with budget constraints.
Convincing users to trust outputs generated by generative AI models, especially in critical applications like healthcare or finance, can be challenging. Establishing trust and acceptance is crucial for the successful integration of generative AI solutions.
Developing and deploying generative AI models often requires a combination of expertise in machine learning, computer science, and domain-specific knowledge. The need for interdisciplinary skills may pose challenges for organizations lacking diverse expertise.
Addressing these restraining factors requires a concerted effort from researchers, developers, policymakers, and industry stakeholders to ensure the responsible and ethical development, deployment, and use of generative AI technologies. Ongoing research and collaboration are essential to overcome these challenges and unlock the full potential of generative AI.
Competitive Landscape
The generative AI landscape is undergoing rapid transformation, propelled by advancements from industry frontrunners, as highlighted by the IMARC Group. Presently, major market players are at the forefront of innovation, introducing novel models, refining existing ones, and employing avant-garde techniques to elevate the quality and diversity of generated content. Notably, investments in research and development are directed towards enhancing image and video synthesis, with applications spanning virtual reality, gaming, content creation, and special effects.
In addition to pioneering technological advancements, key players in the generative AI arena are actively working towards democratizing access to this cutting-edge technology. Initiatives include the development of user-friendly tools, platforms, and APIs designed to empower developers, researchers, and businesses, ensuring seamless integration and utilization of generative AI capabilities. Furthermore, strategic collaborations through partnerships and mergers and acquisitions are a focal point for market leaders, strategically fortifying their positions in this dynamic landscape.
Among the notable entities shaping the generative AI market are:
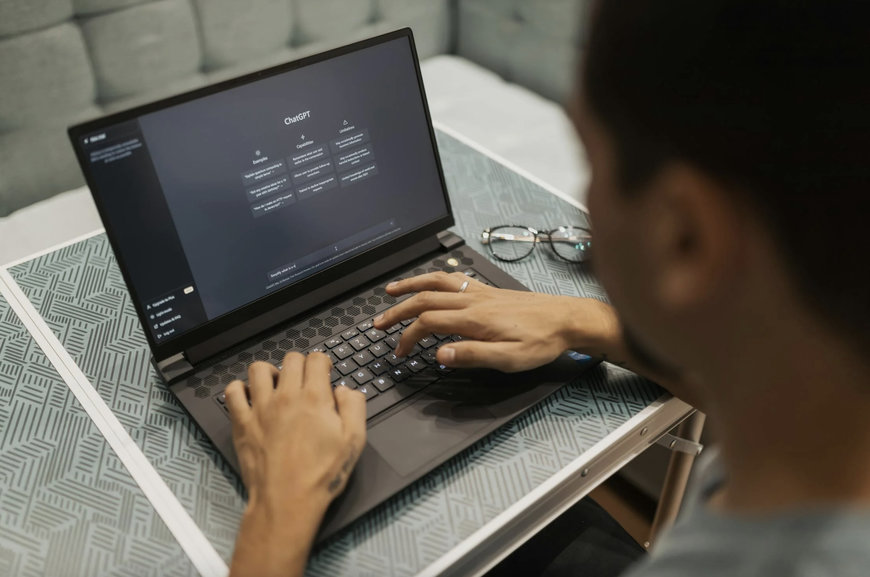
Photo: Matheus Bertellii on Pexels
Restraining factors in Generative AI
While generative AI has experienced substantial growth and advancement, there are also several restraining factors and challenges that impact its widespread adoption.
Generative AI models often require large amounts of data for training, which raises concerns about data privacy and security. The use of personal or sensitive data in training sets may lead to privacy breaches and ethical issues. The potential for misuse of generative AI, especially in the creation of deepfakes or other malicious content, raises ethical concerns. Addressing the responsible and ethical use of these technologies is crucial to mitigate negative consequences.
Generative AI models can inadvertently learn and perpetuate biases present in the training data. This can lead to biased outputs, reinforcing existing societal prejudices and discrimination. Ensuring fairness and addressing bias in generative models is an ongoing challenge.
Many generative AI models, especially complex neural networks, lack interpretability. Understanding how these models generate specific outputs can be challenging, limiting their trustworthiness in critical applications where interpretability is essential.
Training and running sophisticated generative AI models often require significant computational resources, including powerful GPUs and TPUs. This resource intensiveness can be a barrier for smaller organizations or those with limited computing infrastructure.
The legal landscape surrounding generative AI, particularly in the context of intellectual property rights and ownership of generated content, is still evolving. Clear regulatory frameworks are needed to address legal challenges associated with generative AI.
Generative AI models may face challenges in generalizing well to diverse and unseen data. Overfitting to specific patterns in the training data may result in poor performance on new and varied inputs.
Understanding and predicting the behaviour of generative AI models can be difficult. This lack of understanding may hinder the adoption of these models in critical applications where predictability is essential.
Building and maintaining state-of-the-art generative AI models can be expensive. This cost factor may limit the accessibility of generative AI technologies for smaller businesses and organizations with budget constraints.
Convincing users to trust outputs generated by generative AI models, especially in critical applications like healthcare or finance, can be challenging. Establishing trust and acceptance is crucial for the successful integration of generative AI solutions.
Developing and deploying generative AI models often requires a combination of expertise in machine learning, computer science, and domain-specific knowledge. The need for interdisciplinary skills may pose challenges for organizations lacking diverse expertise.
Addressing these restraining factors requires a concerted effort from researchers, developers, policymakers, and industry stakeholders to ensure the responsible and ethical development, deployment, and use of generative AI technologies. Ongoing research and collaboration are essential to overcome these challenges and unlock the full potential of generative AI.
Competitive Landscape
The generative AI landscape is undergoing rapid transformation, propelled by advancements from industry frontrunners, as highlighted by the IMARC Group. Presently, major market players are at the forefront of innovation, introducing novel models, refining existing ones, and employing avant-garde techniques to elevate the quality and diversity of generated content. Notably, investments in research and development are directed towards enhancing image and video synthesis, with applications spanning virtual reality, gaming, content creation, and special effects.
In addition to pioneering technological advancements, key players in the generative AI arena are actively working towards democratizing access to this cutting-edge technology. Initiatives include the development of user-friendly tools, platforms, and APIs designed to empower developers, researchers, and businesses, ensuring seamless integration and utilization of generative AI capabilities. Furthermore, strategic collaborations through partnerships and mergers and acquisitions are a focal point for market leaders, strategically fortifying their positions in this dynamic landscape.
Among the notable entities shaping the generative AI market are:
- Adobe Inc. has been at the forefront of creative software and tools for decades. Their investment in generative AI is evident through products like Adobe Sensei, which leverages AI to enhance user experiences, automate tasks, and generate content. Its integration of generative AI into its suite of creative applications empowers designers, marketers, and content creators to produce personalized, high-quality content efficiently.
- Amazon Web Services Inc. (AWS) provides cloud-based infrastructure and services, including machine learning and AI capabilities. Their SageMaker platform enables developers to build, train, and deploy generative AI models. AWS democratizes access to AI tools, making it easier for businesses to adopt generative AI solutions without heavy upfront investments.
- D-ID specializes in privacy protection through AI. Their technology anonymizes facial images while preserving their utility. In an era of privacy concerns, D-ID’s generative AI solutions are crucial for safeguarding sensitive data, especially in industries like healthcare and finance.
- Google LLC’s research and development in generative AI have led to breakthroughs like DeepDream and BERT. They also offer tools like TensorFlow and AutoML. Google’s open-source contributions and AI-driven products influence the entire industry. Their generative models enhance search, language understanding, and content creation.
- MOSTLY AI Inc. focuses on synthetic data generation. Their platform creates privacy-preserving synthetic datasets for training AI models. Synthetic data is essential for training robust generative models while protecting user privacy. MOSTLY AI’s approach addresses data scarcity and privacy concerns.
- OpenAI is renowned for its research in natural language processing (NLP) and generative models. Their GPT series (including ChatGPT) has transformed how we interact with AI-generated content.OpenAI’s democratization of large-scale language models has sparked innovation across industries, from chatbots to content creation.
- Rephrase.ai specializes in video synthesis using AI. Their technology generates realistic video content from text descriptions. Video content is increasingly vital for marketing, entertainment, and communication. Rephrase.ai’s solutions streamline video production.
- Synthesia offers AI-driven video production tools. Their platform enables automatic video creation by converting text into lifelike videos.The company’s technology accelerates video content production, making it accessible to businesses and creators. Specific data related to Synthesia’s market share is not explicitly mentioned, but their impact aligns with the broader generative AI market trends.
Other Key Industry Developments
Our exploration into the expansive domain of generative AI extends beyond the central focus on leading market participants, encompassing a broader spectrum of pivotal advancements. In this segment, we delve into 'Other Key Industry Developments,' revealing a rich tapestry of fresh ideas, strategic partnerships, and emerging trends that are leaving an indelible mark on the trajectory of generative AI. From groundbreaking research projects to transformative alliances and technological milestones, the evolving landscape unfolds with each noteworthy endeavour.
In June 2023, Salesforce made a significant stride by introducing two generative AI products tailored for the commerce experience and personalized consumer engagement—Commerce GPT and Marketing GPT. The latter, Marketing GPT, harnesses real-time data from Salesforce's cloud platform to generate innovative audience segments, personalized emails, and refined marketing strategies.
Similarly, in the same month, Accenture and Microsoft forged a collaborative effort to empower companies in transforming their businesses through the accelerated potential of generative AI driven by the cloud. This alliance is designed to guide customers in responsibly integrating and extending technology to suit their unique business needs.
May 2023 witnessed SAP SE joining forces with Microsoft to address fundamental business challenges through the incorporation of the latest enterprise-ready innovations. This strategic integration is poised to usher in new experiences, enhancing how businesses attract, retain, and optimize the quality of their workforce.
In the realm of startup acceleration, April 2023 marked a milestone as Amazon Web Services, Inc. unveiled the global Generative AI Accelerator. This initiative provides startups with access to impactful AI tools and models, optimization of machine learning stacks, customized go-to-market strategies, and more.
Lastly, the collaborative efforts of Adobe and NVIDIA in March 2023 stand as a testament to the industry's commitment to advancing generative AI and fostering sophisticated creative workflows. This partnership aims to innovate advanced AI models seamlessly integrated into applications widely used by significant developers and markets, charting a course for the future of generative AI.
Generative AI in 2024
Let's now cast our gaze into the crystal ball of generative AI and catch a glimpse of what the landscape holds for us in 2024.
In the ever-evolving landscape of artificial intelligence, the advent of advanced generative models marks a pivotal moment. As we stand on the cusp of innovation, GPT-4, the successor to the groundbreaking GPT-3, promises to propel us into uncharted territories of natural language understanding, creative expression, and interactive capabilities. GPT-4 is not confined to the realm of text alone. The upcoming generation of language models is set to transcend boundaries, seamlessly integrating multimodal inputs such as images, audio, and video. This expansion will usher in a new era of immersive and expressive interactions, pushing the boundaries of what AI can achieve.
The democratization of artificial intelligence is underway with the rise of fine-tuning tools. Enabling users to tailor pre-trained models to specific tasks, these tools are becoming commonplace. The ability to customize AI empowers businesses and individuals alike, fostering a new era of adaptability to unique needs. Domain-specific models will further enrich this customization trend, offering specialized solutions for fields such as law, medicine, and science. The fine-tuning of models for niche applications promises to enhance performance and relevance in specific domains.
As AI continues its ascent, the need for ethical considerations becomes paramount. Efforts to mitigate bias in generative models will intensify, with a strong focus on fairness, transparency, and accountability. The interpretability of AI models will also see significant advancements, employing techniques like attention maps and rule-based explanations to demystify their decision-making processes.
The collaboration between human creativity and AI is set to reach new heights. Artists, musicians, and writers will join forces with AI to produce innovative works across various mediums. Expect AI-generated music, paintings, and literature to grace galleries and concert halls, showcasing the symbiotic relationship between human ingenuity and artificial intelligence. Tools facilitating style transfer will proliferate, allowing users to seamlessly blend artistic styles. Imagine Picasso-inspired selfies or engaging in conversations with Shakespearean chatbots, blurring the lines between human and machine-generated creativity.
In the realm of healthcare, generative models are poised to revolutionize drug discovery. Predicting molecular structures, simulating protein interactions, and optimizing drug candidates will be accelerated by these advanced AI systems. Medical imaging, powered by AI-generated visuals, will play a crucial role in diagnosis, treatment planning, and disease monitoring.
The future of content creation is automated, with AI taking the reins in writing articles, blog posts, and marketing copy. Personalized experiences will become the norm, with AI delivering tailored news summaries, advertisements, and dynamic website content. Conversational AI, in the form of chatbots and virtual assistants, will evolve to become more human-like, handling diverse tasks from customer queries to emotional support.
Realistic video generation is on the horizon, with tools like DALL-E paving the way for AI-generated video content. This evolution will transform advertising, entertainment, and education. However, ethical challenges loom, requiring robust detection methods and clear ethical guidelines to address the rise of deepfakes.
AI-generated environments will redefine the gaming landscape, providing increasingly realistic virtual worlds for gaming, training simulations, and architectural visualization. Non-player characters (NPCs) will possess dynamic personalities and lifelike behaviour, enhancing the overall gaming experience.
In a harmonious blend of human and AI capabilities, we will witness more projects where AI assists human creativity. Musicians composing alongside AI, writers brainstorming with language models—the possibilities are endless, representing a harmonious fusion of human intuition and AI capabilities.
The future of AI is decentralized, with on-device generative AI gaining prominence. Models running directly on devices, from phones to IoT devices, will address privacy concerns and mark a paradigm shift in how AI is deployed. Synthetic data generated by these models will further contribute to training while preserving individual privacy. As we navigate this dynamic landscape, one thing is certain: the future is full of surprises. Generative AI is at the forefront of shaping our world in ways we cannot fully predict. So, buckle up for an exciting journey into the AI-driven future!
In June 2023, Salesforce made a significant stride by introducing two generative AI products tailored for the commerce experience and personalized consumer engagement—Commerce GPT and Marketing GPT. The latter, Marketing GPT, harnesses real-time data from Salesforce's cloud platform to generate innovative audience segments, personalized emails, and refined marketing strategies.
Similarly, in the same month, Accenture and Microsoft forged a collaborative effort to empower companies in transforming their businesses through the accelerated potential of generative AI driven by the cloud. This alliance is designed to guide customers in responsibly integrating and extending technology to suit their unique business needs.
May 2023 witnessed SAP SE joining forces with Microsoft to address fundamental business challenges through the incorporation of the latest enterprise-ready innovations. This strategic integration is poised to usher in new experiences, enhancing how businesses attract, retain, and optimize the quality of their workforce.
In the realm of startup acceleration, April 2023 marked a milestone as Amazon Web Services, Inc. unveiled the global Generative AI Accelerator. This initiative provides startups with access to impactful AI tools and models, optimization of machine learning stacks, customized go-to-market strategies, and more.
Lastly, the collaborative efforts of Adobe and NVIDIA in March 2023 stand as a testament to the industry's commitment to advancing generative AI and fostering sophisticated creative workflows. This partnership aims to innovate advanced AI models seamlessly integrated into applications widely used by significant developers and markets, charting a course for the future of generative AI.
Generative AI in 2024
Let's now cast our gaze into the crystal ball of generative AI and catch a glimpse of what the landscape holds for us in 2024.
In the ever-evolving landscape of artificial intelligence, the advent of advanced generative models marks a pivotal moment. As we stand on the cusp of innovation, GPT-4, the successor to the groundbreaking GPT-3, promises to propel us into uncharted territories of natural language understanding, creative expression, and interactive capabilities. GPT-4 is not confined to the realm of text alone. The upcoming generation of language models is set to transcend boundaries, seamlessly integrating multimodal inputs such as images, audio, and video. This expansion will usher in a new era of immersive and expressive interactions, pushing the boundaries of what AI can achieve.
The democratization of artificial intelligence is underway with the rise of fine-tuning tools. Enabling users to tailor pre-trained models to specific tasks, these tools are becoming commonplace. The ability to customize AI empowers businesses and individuals alike, fostering a new era of adaptability to unique needs. Domain-specific models will further enrich this customization trend, offering specialized solutions for fields such as law, medicine, and science. The fine-tuning of models for niche applications promises to enhance performance and relevance in specific domains.
As AI continues its ascent, the need for ethical considerations becomes paramount. Efforts to mitigate bias in generative models will intensify, with a strong focus on fairness, transparency, and accountability. The interpretability of AI models will also see significant advancements, employing techniques like attention maps and rule-based explanations to demystify their decision-making processes.
The collaboration between human creativity and AI is set to reach new heights. Artists, musicians, and writers will join forces with AI to produce innovative works across various mediums. Expect AI-generated music, paintings, and literature to grace galleries and concert halls, showcasing the symbiotic relationship between human ingenuity and artificial intelligence. Tools facilitating style transfer will proliferate, allowing users to seamlessly blend artistic styles. Imagine Picasso-inspired selfies or engaging in conversations with Shakespearean chatbots, blurring the lines between human and machine-generated creativity.
In the realm of healthcare, generative models are poised to revolutionize drug discovery. Predicting molecular structures, simulating protein interactions, and optimizing drug candidates will be accelerated by these advanced AI systems. Medical imaging, powered by AI-generated visuals, will play a crucial role in diagnosis, treatment planning, and disease monitoring.
The future of content creation is automated, with AI taking the reins in writing articles, blog posts, and marketing copy. Personalized experiences will become the norm, with AI delivering tailored news summaries, advertisements, and dynamic website content. Conversational AI, in the form of chatbots and virtual assistants, will evolve to become more human-like, handling diverse tasks from customer queries to emotional support.
Realistic video generation is on the horizon, with tools like DALL-E paving the way for AI-generated video content. This evolution will transform advertising, entertainment, and education. However, ethical challenges loom, requiring robust detection methods and clear ethical guidelines to address the rise of deepfakes.
AI-generated environments will redefine the gaming landscape, providing increasingly realistic virtual worlds for gaming, training simulations, and architectural visualization. Non-player characters (NPCs) will possess dynamic personalities and lifelike behaviour, enhancing the overall gaming experience.
In a harmonious blend of human and AI capabilities, we will witness more projects where AI assists human creativity. Musicians composing alongside AI, writers brainstorming with language models—the possibilities are endless, representing a harmonious fusion of human intuition and AI capabilities.
The future of AI is decentralized, with on-device generative AI gaining prominence. Models running directly on devices, from phones to IoT devices, will address privacy concerns and mark a paradigm shift in how AI is deployed. Synthetic data generated by these models will further contribute to training while preserving individual privacy. As we navigate this dynamic landscape, one thing is certain: the future is full of surprises. Generative AI is at the forefront of shaping our world in ways we cannot fully predict. So, buckle up for an exciting journey into the AI-driven future!